神经网络在模糊Petri网故障诊断中的应用
来源:岁月联盟
时间:2010-08-30
1 引言
Petri网与模糊理论相结合,以及Petri网与神经网络相结合已经在故障诊断过程中得到了应用,虽然模糊Petri网具有很强的模糊推理能力,但是其缺乏较强的自学习能力,该缺点严重制约了模糊Petri网在各个领域的应用,所以将人工神经网络引入到模糊Petri网中,建立故障诊断的模糊神经网络Petri 网模型,提高了故障诊断系统的精度。本文以船舶主机燃油子系统过程为例,利用模糊神经网络Petri网对该过程中的故障诊断进行建模。2 模糊神经Petri网模型
2. 1 模糊神经Petri网网定义
模糊神经Petri网定义为一个十二元组,

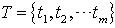

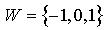


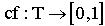



2. 2 模糊神经Petri网模型的学习功能
模糊神经Petri网(参见图1),具有神经网络的某些特性,因此可先用神经网络的学习算法进行训练,再调整网络结构中的权值,从而获取知识。图2为模糊神经Petri网转化为神经元模型。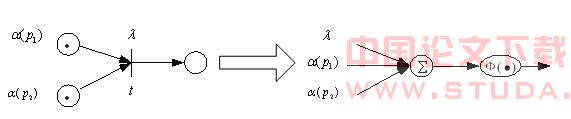
3 应用实例
3.1 生产过程
船舶主柴油机是机舱系统最为关键的设备,也是一种非常复杂的动力装置系统。它既是一个由往复式机械与其他机械构成的动力装置系统,也包括了由控制装置、伺服系统、检测与显示仪表以及安全保护和报警系统等组成的电气控制系统,同时它还是一个复杂的热工过程。由于船舶主机的极端重要性,其故障诊断和工矿监视问题历来深受重视。目前人们至少已从三个不同的方面探索研究了船舶主机的故障诊断问题,其一是振动分析,其二是通过润滑油品质指标的分析来判断主机机械磨损情况,其三就是从热工参数出发诊断船舶主机系统的故障。本文利用第三种方法,选用燃油流量 、燃油压力 和燃油黏度 ,诊断燃油子系统故障。其流程图如图3所示。3.2 模糊神经Petri网模型
船舶主机燃油子系统故障诊断的模糊神经Petri网模型如图4所示。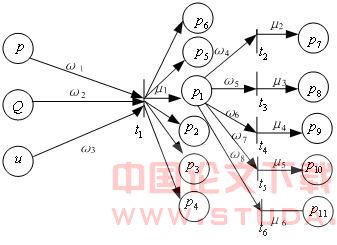

输入 | 输出 | 故 障原 因 | |||||
P1 | X | P7 | P8 | P9 | P10 | P11 | |
0 | X | 1 | 0 | 0 | 0 | 0 | 正常 |
1 | X1 | 0 | 1 | 0 | 0 | 0 | 超负荷 |
1 | X2 | 0 | 0 | 1 | 0 | 0 | 后燃 |
1 | X3 | 0 | 0 | 0 | 1 | 0 | 提前着火 |
1 | X4 | 0 | 0 | 0 | 0 | 1 | 燃油管路泄露 |
表2 燃油子系统的故障诊断实例
序号 | 输入 | 输出(%) | ||||||||
Q | P | u | P0 | P1 | P2 | P3 | P4 | P5 | P6 | |
1 | H | N | N | 3 | 96 | 0 | 0 | 0 | 1 | 0 |
2 | H | 0.6N | 0.6N | 9 | 74 | 1 | 9 | 2 | 3 | 2 |
3 | H | -0.4N | -0.4N | 4 | 76 | 0 | 0 | 0 | 20 | 0 |
4 | 1.2H | -0.4N | -0.4N | 1 | 79 | 0 | 0 | 0 | 20 | 0 |
5 | 0.8H | -0.4N | -0.4N | 9 | 69 | 0 | 0 | 0 | 21 | 1 |
序号 | 输入 | 输出(%) | ||||
P1 | X | P8 | P9 | P10 | P11 | |
1 | 1 | X1 | 97 | 3 | 0 | 0 |
2 | 1 | X2 | 1 | 93 | 6 | 0 |
3 | 1 | X3 | 0 | 4 | 90 | 6 |
4 | 1 | X4 | 0 | 0 | 8 | 92 |
5 | 1 | X5 | 68 | 32 | 0 | 0 |
5 结束语
本文将人工神经网络引入到模糊Petri网中,并以船舶主机燃油子系统过程为例,对过程中存在的故障进行诊断,通过实例说明该方法可以提高故障诊断系统的精度。[1] 胡志刚等,基于模糊神经Petri网的故障诊断模型[J].小型微型机系统,2005,11(26)[2] 王磊等,甲醇合成过程中基于MAS的故障诊断方法[J].华东理工大学学报,2006,1(32)[3] Korie m S M. A fuzzy Petri net tool for modeling and verification of knowledge-based systems[J]. The Computer Journal,2000,43(3):206-223[4] 大卫 R,奥兰 H.(黄建文,赵不贿译)佩特利网和逻辑控制器图形表示工具 (GRAFACET) [M]. 北京:机械出版社,1996[5] Ahson S I. Petri Net Models of Fuzzy Neural Networks. IEEE Trans on SMC, 1995, 25(6): 926-932[6] 韩光臣等,基于模糊概率Petri网系统的故障诊断仿真研究.计算机集成制造系统.2006,4(12)[7] Tsang E C C,Yeung D S, Lee J W T. Learning capability in fuzzy Petri net[J]. In Proceeding of the 1999 IEEE International Conference on Systems,Man,and Cybernetics,Tokyo, 1999:355-360[8] J. IE BAIL, H. ALLA, R. DAVID, “Hybrid Petri Nets”,European Control Conference, Grenoble (F), July 199 1,pp. 1472-1477[9] Champagnat.R, Estebah.P, Pingabld.H, etc. Petri net based modeling of hybrid systems. Computers In Industry, 36(1998): 139-146上一篇:基于语义网的知识管理研究
下一篇:管网水水质预测及其二次污染研究